Using AI and machine learning to predict weather patterns and diagnose crop diseases in the Sahel region
This week, we spotlight how Dr Chimango Nyasulu, a recent Regional Scholarship and Innovation Fund (Rsif) supported PhD graduate from Malawi, is using AI and machine learning to predict weather patterns and diagnose crop diseases in the Sahel region. Dr Nyasulu is addressing agricultural challenges in the Sahel region through innovative machine learning research. He has made significant strides in addressing agricultural challenges posed by climate change in the Sahel region. His innovative research at Gaston Berger University of Saint Louis, Senegal, focused on using machine learning to predict weather patterns and diagnose crop diseases, aiming to enhance agricultural resilience and productivity.
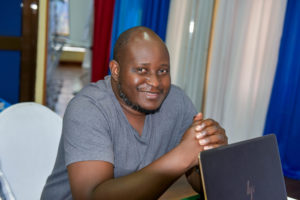
Climate change brings numerous challenges to agriculture in the Sahel, including unpredictable rainfall, rising temperatures, and increased incidence of pests and diseases. To help farmers cope with these challenges, Dr Nyasulu’s research explored the effectiveness of machine learning techniques in forecasting key weather variables and classifying crop diseases.
Dr Nyasulu’s study used various machine learning methods to forecast daily rainfall, relative humidity, maximum temperature, and minimum temperature in Senegal. The stacked Ensemble Model proved to be the most accurate, outperforming other techniques by accurately predicting weather patterns based on spatial distribution and annual cycles. The use of polynomial features improved the model’s performance, reducing errors and increasing accuracy for daily rainfall forecasting.
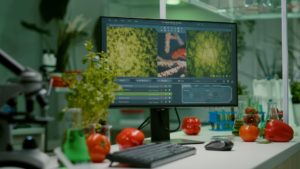
In addition to weather prediction, Dr Nyasulu focused on classifying and quantifying the severity of tomato leaf fungal diseases using machine learning classifiers. Techniques like Support Vector Machine, Random Forest, K-Nearest Neighbors, and Artificial Neural Networks were applied to identify diseases such as Alternaria, Curvularia, Helminthosporium, and Lasiodiplodia based on texture features. The Artificial Neural Network achieved an impressive 91% accuracy rate. Further, transfer learning with Convolutional Neural Networks, specifically MobileNet, enhanced the classification accuracy to 96.3%.
The practical application of Dr Nyasulu’s research led to the development of a prototype called E-FarmAI, integrating the Ensemble Model for weather forecasting and MobileNet for disease classification. This tool has the potential to significantly boost food security in Senegal and other African nations by providing farmers with accurate weather predictions and effective disease management strategies.
Dr Nyasulu’s research holds valuable insights for policymakers, researchers, and development organizations aiming to promote agricultural productivity and resilience in Africa. Currently, he continues his work as a Researcher in Artificial Intelligence in the ICT Department of Mzuzu University in Malawi, contributing to the advancement of AI solutions for agricultural challenges.
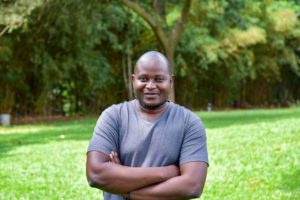
The Rsif program has played a crucial role in supporting Dr Nyasulu and other students, fostering innovation and practical solutions to real-world problems in sub-Saharan Africa. His work exemplifies how Rsif students are solving pressing African problems, driving positive impacts on their countries and the broader sub-Saharan region. Rsif’s contribution to strengthening higher education and scientific research is vital for the continent’s sustainable development and building resilience to climate change.